Truckin' Through Time: Building a Grateful Dead Setlist Database
Introduction
Using Python, I constructed a Grateful Dead setlist archive (1970s-1990s) from abandoned webpages. This post covers my first experience writing a web scraper to aggregate the Grateful Dead setlist data from Mark Leone’s old Carnegie Mellon website .
Mark was, I believe, a researcher at CMU. I didn’t delve deeply into Mark’s official role or position. My apologies to Mark if he happens to be reading this! Nonetheless, I appreciate his efforts in aggregating this data; it greatly contributed to the project.
Tools and Technologies Used:
- Python
- BeautifulSoup
- SQLModel
- Ollama (LLama3-8B)
- SQLite
You can find all the code and the SQLite database containing the data I scraped on my GitHub .
The Inspiration
A few days ago, I was out on a walk, brainstorming the kind of dataset I wanted to use to learn more about data processing libraries in Python. Then, “Uncle John’s Band” by the Grateful Dead shuffled on, sparking the idea to explore a Grateful Dead dataset. While I wouldn’t call myself a Dead Head 💀, the band’s extensive touring history and tendency to record their shows always intrigued me.
I began diving into the depths of Google, searching for Grateful Dead setlist databases, and encountered these long-forgotten websites from the 1990s and early 2000s that enthusiasts had spent considerable time putting together. One such site, created by Mark Leone , a researcher at Carnegie Mellon University, stood out to me because it looked fairly easy to scrape for data. While I’m sure there are beautifully curated and well-structured datasets out there with the same information, I embarked on this journey of writing a web scraper to create my own database from Mark Leone’s old site at Carnegie Mellon . This old website, still hosted on Carnegie Mellon’s Computer Science Department’s website , includes a bunch of random Grateful Dead things, including setlists from Grateful Dead tours from 1972-1995 .
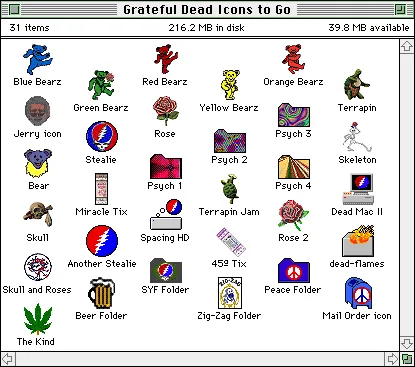
Vintage Grateful Dead Mac Icons obtained from Mark Leone’s old Carnegie Mellon website . I spent a little too much time looking at all the items in the Apache Directory listing … 😅
Project Overview
Before we dive into the technical details, here’s a flowchart of how my simple program works to create this Grateful Dead setlist database:
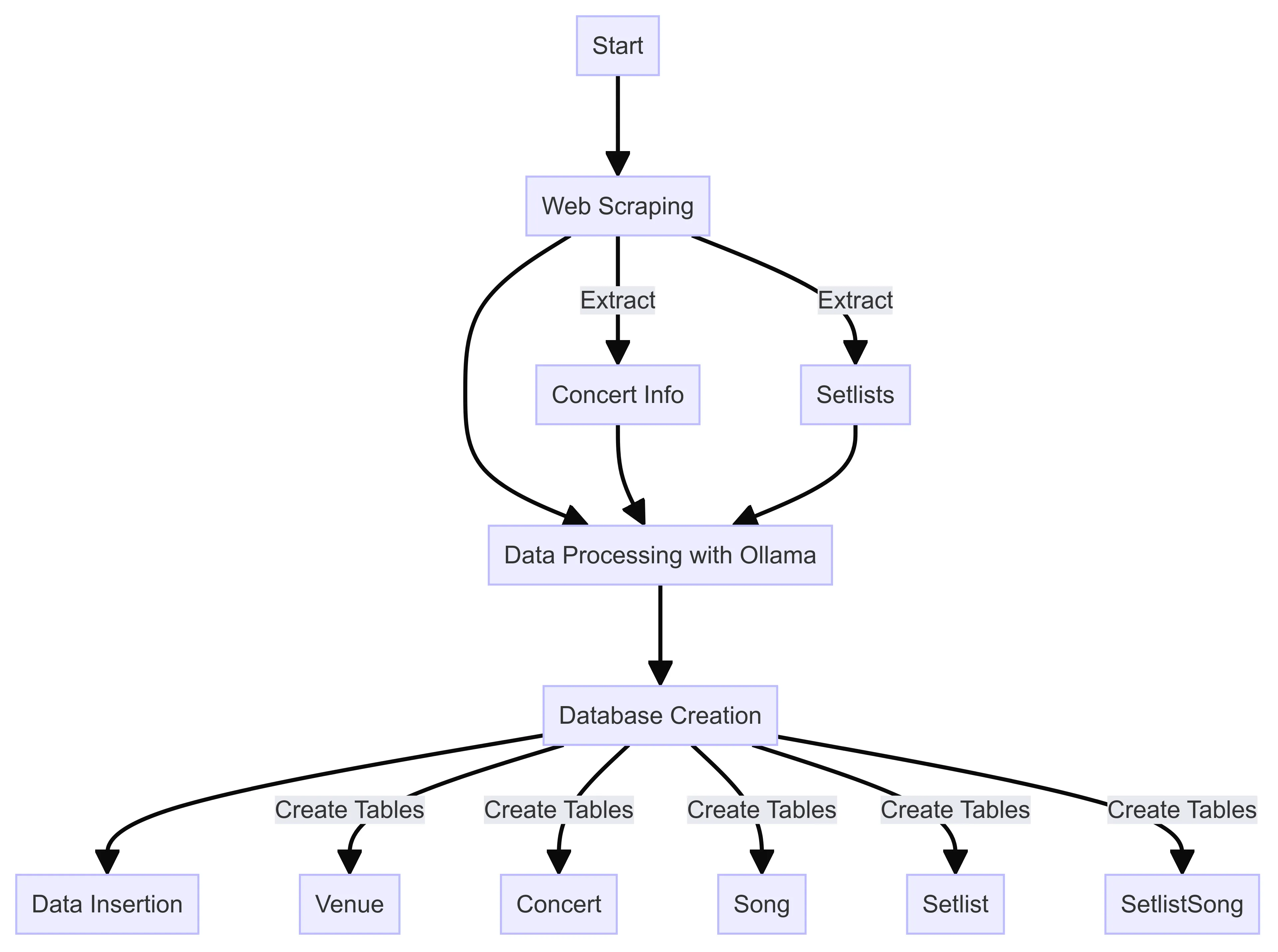
Flowchart outlining the main steps of the Grateful Dead setlist database creation process
This flowchart outlines the main steps of the program I wrote. The key stages of this process are:
- Data Extraction with Web Scraping: Gathering raw setlist data from abandoned webpages.
- Data Processing/Parsing: Using Ollama (Llama3) to structure the raw data into a usable format.
- Persisting the Data: Storing the processed information in a SQLite database.
This rest of this post details each of these steps. This approach allowed me to transform unstructured web data into a fairly comprehensive, queryable database of Grateful Dead performances.
Web Scraping
Web scraping is a general technique used to extract information from websites. In this project, I scraped setlist data from Mark Leone’s CMU website, which archived concert setlists of the Grateful Dead. The goal was to compile a comprehensive and structured dataset of these setlists for analysis.
Why Web Scraping?
The data on the website was not available in a format suitable for analysis. By scraping the website, I transformed this rich, unstructured data into a structured format.
The Information Architecture
The data on Mark Leone’s CMU website was organized with a setlist “home” page linking to concerts by year. Each year’s page included links to text files with the setlists.
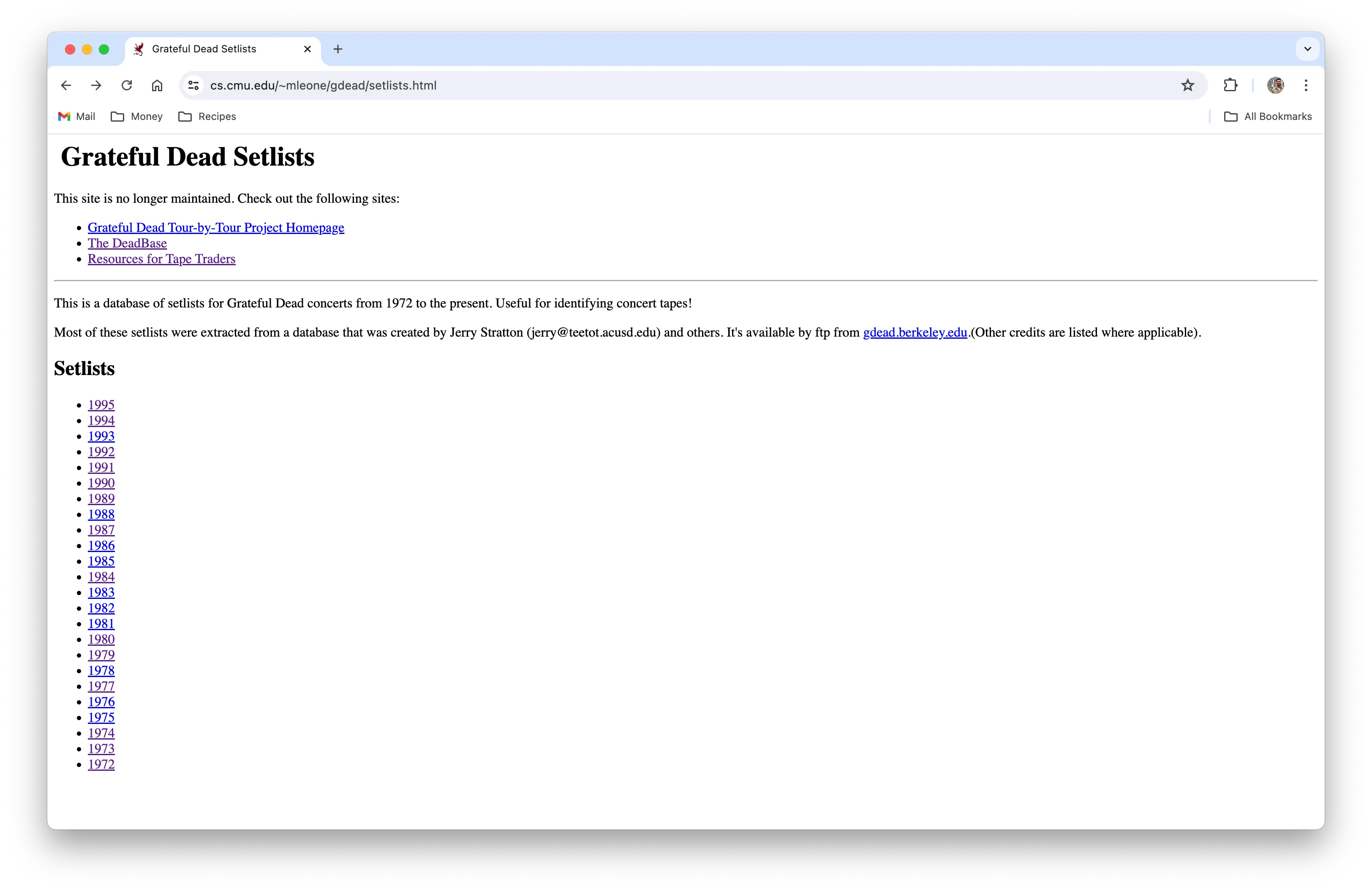
The “home” page with links to concerts from each specific year.
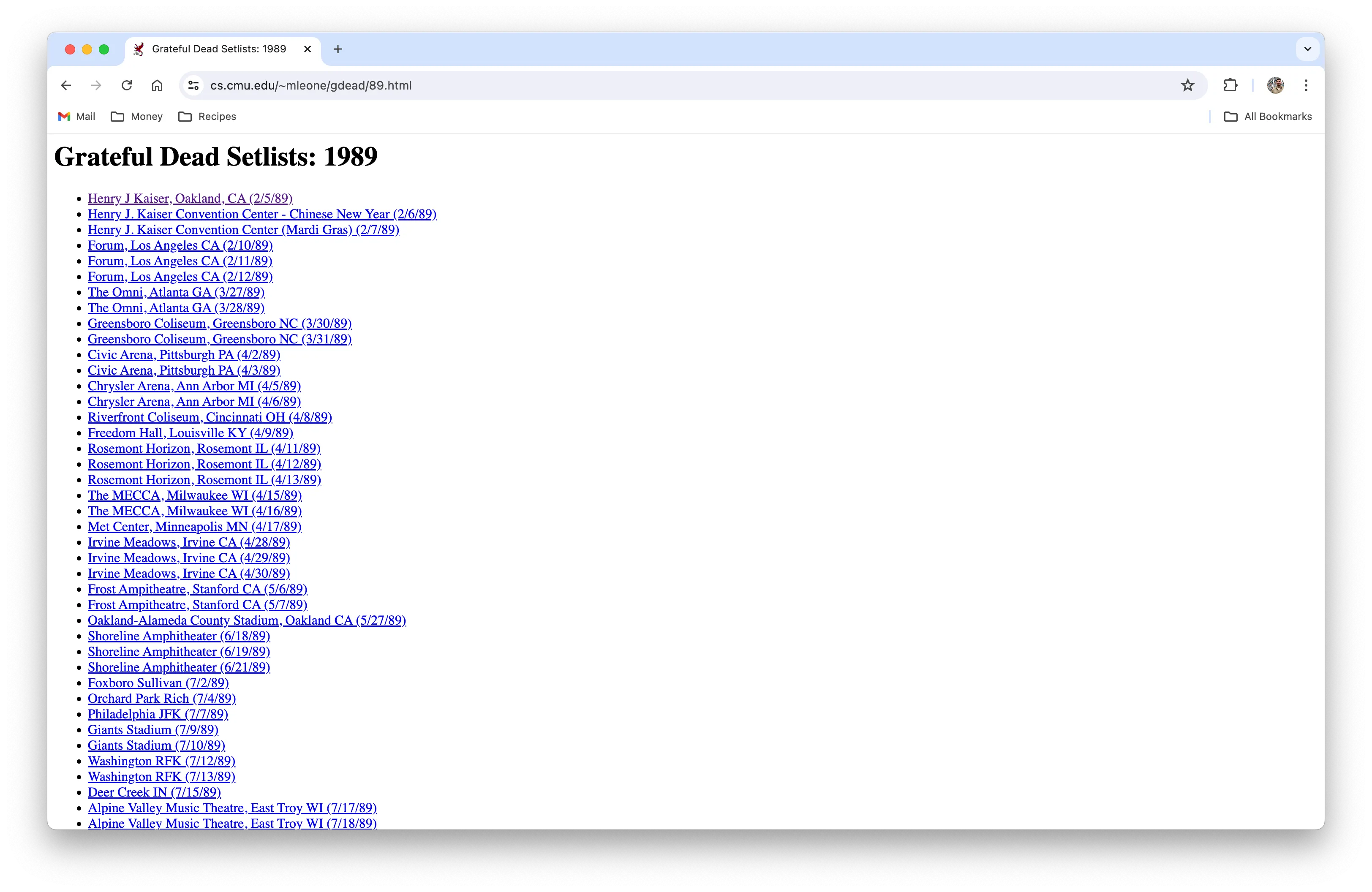
The concert list for a 1989 with links to setlists for each concert.
The text files were mostly structured, though not always consistently - more on how I handled that later on this post.
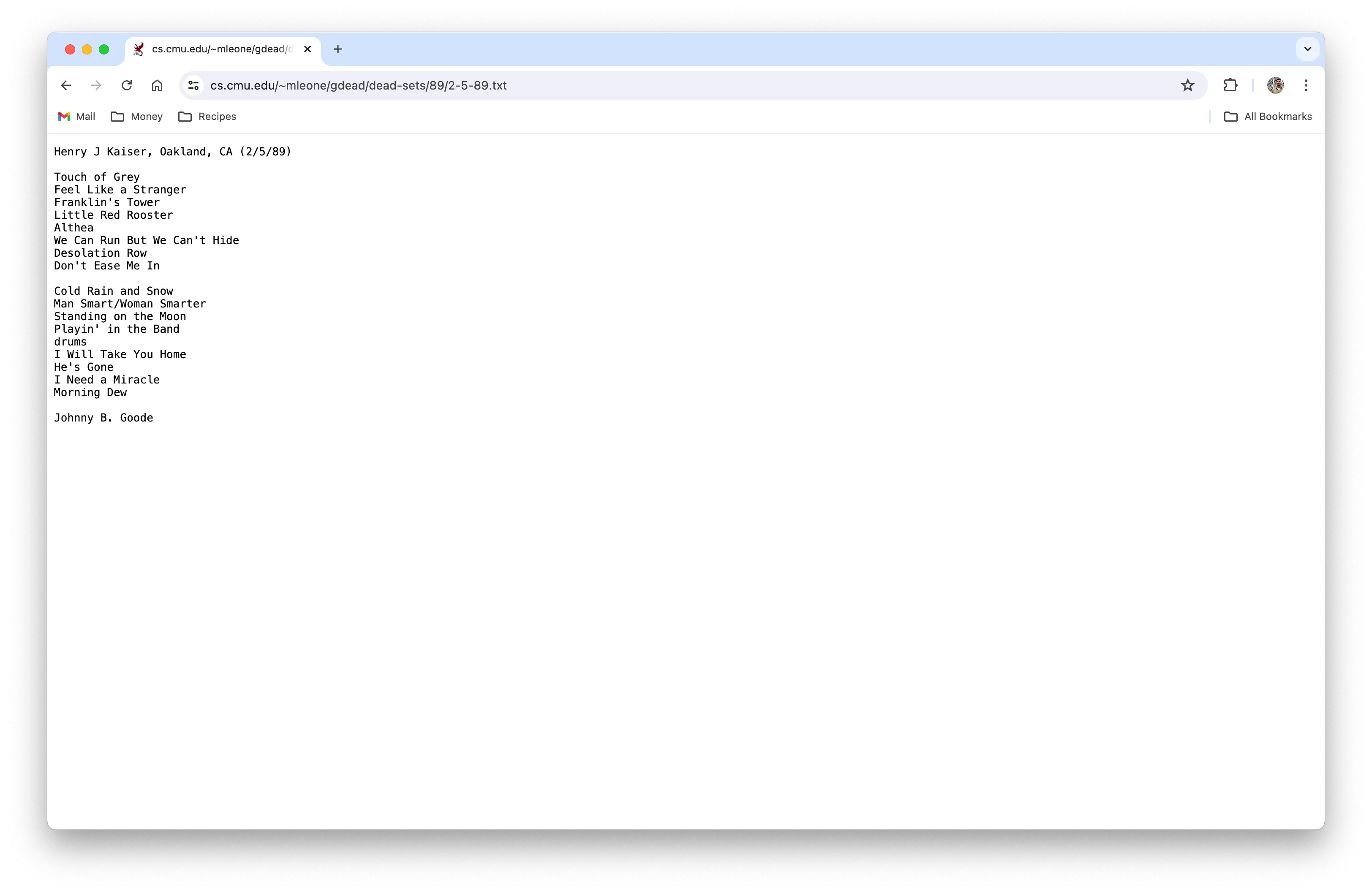
The setlist for a show at Henry J Kaiser Convention Center in Oakland, CA on 2/5/89.
Using BeautifulSoup
To scrape the setlist data, I used Python’s BeautifulSoup library to parse the HTML of the webpages. The code iterates through the “home” page, fetching links for each touring year. For each year, it retrieves information on individual concerts and their setlists, storing everything in structured objects.
Here’s the a code snippet containing the bulk of my BeautifulSoup usage:
ROOT_URL = "https://www.cs.cmu.edu/~mleone/gdead/"
def get_touring_years() -> list[TouringYear]: logger.info("Fetching yearly setlists...") response = requests.get(f"{ROOT_URL}/setlists.html") if not response.ok: logger.fatal(f"Unable to yearly setlists. Status Code: {response.status_code}") sys.exit(1) soup = BeautifulSoup(response.text, "html.parser") # The second ul (list) on that setlist "home" page contains the touring year links touring_year_links = soup.find_all("ul")[1].find_all("a")
touring_years = [ TouringYear(year=link.text, relative_url=link["href"]) for link in touring_year_links ] for touring_year in touring_years: logger.info(f"Getting setlists for {touring_year.year}") url = f"{ROOT_URL}/{touring_year.relative_url}" response = requests.get(url) if not response.ok: logger.fatal( f"Unable to get setlists for year {touring_year.year}. Status Code: {response.status_code}" ) sys.exit(1) soup = BeautifulSoup(response.text, "html.parser") concert_links = soup.find_all("ul")[0].find_all("a") setlists = [ ConcertData( touring_year=touring_year, name=link.text.strip(), relative_url=link["href"], ) for link in concert_links ]
touring_year.concerts = setlists return touring_years
TouringYear
and ConcertData
are just simple
Pydantic models used to store the scraped
data before processing it further and ultimately writing it to the database.
Extracting Data as JSON with Llama3
Concert and Venue Information
Parsing the concert data presented challenges due to varying formats. Initially, I used regex to extract venue names, cities, states, and countries, but inconsistencies made this difficult.
For example:
- 1972 : Formatted consistently, making regex effective:
Winterland Arena, San Francisco, CA (1/2/72)Academy of Music, New York, NY (3/27/72)Empire Pool, Wembley, XENG (4/8/72)City Hall, Newcastle, XENG (4/11/72)Tivoli Theater, Copenhagen, XDEN (4/15/72)Aarhus University, Aarhus, XDEN (4/16/72)
I managed to write a regex to parse this data, incorporating custom logic to identify international shows by the "X"
prefix in the country code. However, this was only the beginning of the challenges.
- 1976 : Minor variations but still parseable:
Paramount Theatre, Portland, Ore. (6/3/76)Paramount Theatre, Portland, Ore. (6/4/76)Boston Music Hall, Boston, Mass (6/9/76)Boston Music Hall, Boston, Mass. (6/10/76)Boston Music Hall, Boston, Mass. (6/11/76)Boston Music Hall, Boston, Mass. (6/12/76)
- 1987 : Increased complexity with abbreviations and missing details:
HJK (11/6/87)HJK (11/7/87)HJK (11/8/87)Long Beach Arena (11/13/87)Long Beach (11/14/87)Long Beach Arena (11/15/87)Oakland Coliseum Arena (12/27/87)
Regex alone was insufficient for handling these variations (and more). After tweaking the logic several times, I of course tried using ChatGPT to extract the JSON from the setlist. This worked phenomenally.
I considered using OpenAI’s API, which even has a JSON mode to ensure only JSON is returned, but the API is not free, and I didn’t want to pay for something I was just experimenting with.
I explored running other LLMs locally on my MacBook, starting with LMStudio . It worked well, except I couldn’t get the models it was serving to return JSON reliably. Eventually, I found that Ollama provided a solution locally, avoiding any costs. Ollama has a JSON mode that reliably ensures the model response is JSON, simplifying further processing.
Setlist Information
I faced similar issues when extracting setlist information all individually contained in their setlists. The issues stemmed from the data not being consistently formatted. My first naive approach was to loop over all lines in the text files skipping the first line which always seemed to reliably repeat the concert and information I had already processed.
Extracting setlists faced similar inconsistencies. Initially, I looped over text files, skipping the first line, which repeated concert information. Consistent formats, like the show at Theatre Tower in Upper Darby, PA on 6/21/76 was formatted consistently:
Tower Theatre, Upper Darby, PA (6/21/76)
Music Never StoppedSugareeMama TriedBrown Eyed WomenCassidyRow JimmyLooks Like RainScarlet BegoniasLazy Lightnin'SupplicationCandymanPromised Land
The WheelSamson and DelilahHelp on the WaySlipknot!Franklin's TowerIt Must Have Been the RosesDancin' in the StreetsWharf RatAround and Around
Johnny B. Goode
However, some shows such at Delta Center in Salt Lake City, UT on Sunday 2/19/95 required additional processing:
Delta Center, Salt Lake City, UT (Sunday, 2/19/95)
Jack StrawSugareeWalkin' BluesAlabama GetawayMe & My Uncle* ->Big River*Don't Ease Me In*
China Cat Sunflower ->I Know You RiderSamson and DelilahIf the Shoe FitsNew Speedway Boogie ->Estimated Prophet ->Drums ->Space ->I Need A Miracle ->Attics of my Life ->Johnny B. Goode
E: Baby Blue
*Bobby Acoustic*(first Alabama Getaway since 6/18/89)**(soundcheck included Alabama Getaway, Attics, Schoolgirl,and It's a Man's World)*
- The ”->” symbol indicates a song transition.
- Footnotes can span more than 1 line and add extra details for certain songs and/or the setlist.
- The encore song is prepended with “E:“.
Rather than complicating my initial regex approach with additional logic to handle these variations, I used Ollama to structure setlists into JSON, ensuring accuracy.
Data Extraction using Ollama
Using the ollama-python library, which is just a simple wrapper around the Ollama server API, this became a lot simpler than trying to create multiple regex patterns to potentially match all the concert and setlist variations.
I ran the Ollama server on my 2021 M1 Macbook Pro with 16GM RAM serving Llama3-8B .
import datetimeimport jsonimport logging
from httpx import ConnectErrorfrom ollama import Client as OllamaClient
from .prompts import PARSE_CONCERT_PROMPT, PARSE_SETLIST_PROMPT
logger = logging.getLogger(__name__)
class LLMParseError(Exception): """Represents an error that occurred while the LLM was trying parse and extract information out of some given text. """3 collapsed lines
def __init__(self, msg): super().__init__(msg)
class Client: """ A client to interact with the LLM served by Ollama for parsing concert and setlist information. """
def __init__(self, ollama_host: str, model: str):4 collapsed lines
self.model = model self.ollama_url = ollama_host self.client = OllamaClient(host=ollama_host) self._validate_config()
def _validate_config(self):9 collapsed lines
""" Validates the availability of the Ollama server. """ try: self.client.list() except ConnectError: raise RuntimeError( f"Failed to establish a connection to the Ollama server running on {self.ollama_url}" )
def _chat_with_llm(self, prompt: str, prompt_formatters: dict[str, str]) -> dict: try: completion = self.client.chat( model=self.model, messages=[ { "role": "system", "content": ( "You are a text extraction assistant. You will be helping me parse concert" " and setlist information from text files." ), }, { "role": "user", "content": prompt.format(**prompt_formatters), }, ],
format="json", ) content = completion["message"]["content"] return json.loads(content) except json.JSONDecodeError: raise LLMParseError( f"LLM did not return valid JSON: {completion['message']['content']}" ) except Exception as e: raise LLMParseError(str(e))
def parse_setlist(self, setlist: str) -> tuple[list[dict], str]: message = self._chat_with_llm( prompt=PARSE_SETLIST_PROMPT, prompt_formatters={"setlist": setlist} ) songs = message.get("songs", []) notes = message.get("notes", "") return songs, notes
def parse_concert_name( self, concert: str ) -> tuple[str, str, str, str, datetime.date]: message = self._chat_with_llm( prompt=PARSE_CONCERT_PROMPT, prompt_formatters={"concert": concert} ) venue = message.get("venue", "") city = message.get("city", "") state = message.get("state", "") country = message.get("country", "") concert_date_str = message.get("date", "") concert_date = datetime.datetime.strptime(concert_date_str, "%m/%d/%Y").date() return venue, city, state, country, concert_date
You can find this code on GitHub as well.
I provided the LLM with the following prompts providing examples of what structure I wanted the JSON to be returned in.
PARSE_CONCERT_PROMPT = """Given the following Grateful Dead concert information, return to me the following informationin a JSON object. Venue, City, State, Country, Date (in format MM/DD/YYYY).- If country information is not there, use your knowledge based on the concert information to fill it in.- If the concert does not place in the USA, return null for state and just provide the country.
Do not return to me any additional information about what you returned. Only return the JSON object.
Example concert information:
The Spectrum, Philadelphia, PA (4/22/77)
Example JSON Object:
{{ "venue": "The Spectrum", "city": "Philadelphia", "state": "PA", "country": "USA", "date": "04/22/1977"}}
This is the concert you need to parse and return JSON for:
{concert}"""
PARSE_SETLIST_PROMPT = """Given the following text file representing a setlist from a Grateful dead concert, return to theme the following information in a JSON object.- List of songs played and any special notes about the setlist. - Use your own judgement to create the notes. They should be concise. - A song should be represented with the following information: title, order played, and whether it was transition into another song. - Please correct any typos you may find and remove any extraneous information.
Example setlist:
NBC Studios, New York, NY (9/17/87)
Comments: David Letterman
Good Lovin'All Along the WatchtowerWalkin' the DogTore Up Over YouKansas City
Masterpiece
Example JSON Object:
{{ "songs": [ {{ "title": "Good Lovin'", "order": 1, "transition": false }}, {{ "title": "All Along the Watchtower", "order": 2, "transition": false }}, {{ "title": "Walking the Dog", "order": 3, "transition": false }}, {{ "title": "Tore Up Over You", "order": 4, "transition": false }}, {{ "title": "Kansas City", "order": 5, "transition": false }}, {{ "title": "Masterpiece", "order": 6, "transition": false }} ], "notes": "Played on David Letterman Show"}}
Here is the setlist for you to parse:
{setlist}
This is the end of the setlist. Please do not return to me any additional information about what you returned. Only return the JSON object.
The script took approximately 10 hours to run, primarily due to the inference time for the Ollama server, especially when processing the setlist songs. Below is a snippet of the Ollama server logs. The 20.5-second call was for processing the setlist information, while the 2.6-second call was for processing the concert information. These times varied slightly, but on average, the inference time to process and extract the setlist information was about 9-10 times longer.
[GIN] 2024/07/03 - 01:21:33 | 200 | 20.550543084s | 127.0.0.1 | POST "/api/chat"[GIN] 2024/07/03 - 01:21:36 | 200 | 2.627832209s | 127.0.0.1 | POST "/api/chat"
Structuring the Database Tables
To organize the scraped and processed data, I used a Python library called SQLModel . It helps define the structure of our database tables based using Python objects .
This was the schema I ended up creating:
Model | Description |
---|---|
Concert | Represents a concert event with attributes like venue (foreign key to Venue table), date, and a relationship with Setlist model (one concert can have many setlists). |
Venue | Represents a venue with attributes like name, city, state (optional), and country. |
Song | Represents a song with attributes like title and a relationship with SetlistSong model (one song can be played in many setlists). |
Setlist | Represents a setlist for a concert with attributes like concert (foreign key to Concert table), notes, and relationships with both Concert (one concert has many setlists) and SetlistSong model (one setlist can have many songs). |
SetlistSong | Represents a specific song played in a setlist with attributes like setlist (foreign key to Setlist table), song (foreign key to Song table), order (position in the setlist), and transition (boolean indicating if there was a transition between songs). |
Results
When the script completed, I had gathered a good chunk of data. The following are the row counts contained in my database tables I wrote to after processing the concert venue and setlist information.
Table | Count |
---|---|
Venue | 484 |
Concert | 484 |
Song | 564 |
SetlistSong | 31546 |
Setlist | 1604 |
It’s quite usable in its current form.
For example, we can write queries to answer the following questions.
How many times was Truckin’ played?
sqlite> SELECT COUNT(*) AS times_played ...> FROM SetlistSong ...> JOIN Song ON SetlistSong.song_id = Song.id ...> WHERE Song.title = 'Truckin''';times_played------------426
How many shows were not played in the USA?
sqlite> SELECT COUNT(*) AS shows_not_in_usa ...> FROM Concert ...> JOIN Venue ON Concert.venue_id = Venue.id ...> WHERE Venue.country NOT IN ('USA') ...> ;shows_not_in_usa----------------62
How many shows were played each year?
sqlite> SELECT SUBSTRING(date, 1, 4) AS concert_year, COUNT(*) AS number_of_shows ...> FROM Concert ...> GROUP BY SUBSTRING(date, 1, 4) ...> ORDER BY concert_year;concert_year number_of_shows------------ ---------------1972 871973 731974 401975 41976 411977 601978 811979 761980 871981 861982 631983 661984 641985 711986 461987 861988 801989 731990 741991 771992 541993 821994 851995 48
But there were quite a few data quality issues that are going to impact the accuracy of the analysis.
Data Quality Issues
The script I wrote executed without errors, resulting in a sizable database. Despite not including error handling in my code to process date information, the LLM consistently returned dates in the MM/DD/YYYY format. However, this does not mean everything worked perfectly. There are still some data quality issues that the LLM was unable to resolve. Perhaps if I had provided the LLM with previously processed data, it would have produced more consistent outputs.
For instance, consider some of the Grateful Dead concerts from 1987 that were challenging to parse using regex:
HJK (11/6/87)HJK (11/7/87)HJK (11/8/87)Long Beach Arena (11/13/87)Long Beach (11/14/87)Long Beach Arena (11/15/87)
I basically told the LLM in the prompt to use its best judgement when extracting concert details.
sqlite> select id, name, city, state, country from venue where "name" like "%hjk%";188|HJK|||USA189|HJK|unknown||USA
It turns out that “HJK” stands for the Henry J. Kaiser Convention Center located in Oakland, CA. Llama3 didn’t recognize this, so it returned the data inconsistently: sometimes with empty strings for city and state, and other times with “unknown” for the city.
sqlite> select id, name, city, state, country from venue where "city" like "%long%";163|Long Beach Arena|Long Beach|CA|USA190|Long Beach|Long Beach|CA|USA
The show listed as “Long Beach (11/14/87)” should have been written as “Long Beach Arena (11/14/87),” but Llama3 wasn’t able to identify and correct this data issue.
sqlite> select id, title from song where "title" like "%corin%";65|Corinna171|Corina
Bob Dylan’s Corinna that the Grateful Dead covered with him, is spelled with 2 N’s and is actually officially titled “Corinna, Corinna”.
There are plenty more small issues like this that will require more processing to correct.
Takeaways
This project was learning experience for me, even though the database I created isn’t unique. Modern Grateful Dead sites like herbibot.com and setlist.fm already have this data cleaned and well-organized. Here are some of my key takeaways:
- Web Scraping with BeautifulSoup: BeautifulSoup proved to be a very useful library for parsing HTML in Python.
- Data Extraction with LLMs: Using Ollama to extract data into structured JSON is amazing. This approach simplified the extraction process, especially when dealing with inconsistent formats. JSON extraction can be incredibly useful for many tasks, and exploring local LLMs can save costs associated with API usage.
- Handling Data Inconsistencies: Despite the LLM’s capabilities, some data quality issues persisted. This highlights the importance of combining automated tools with manual verification for critical data projects.
- Future Applications: The methods and tools used in this project have broad applications beyond just creating a Grateful Dead setlist database. They can be adapted for various data extraction and structuring tasks in the real world ™.
Overall, this project was interesting and I learned quite a bit. I hope to clean this data up and do some more thoughful analysis on the setlists.